O&M
Why Predictive Reliability Is the Key To Improved Maintenance Performance
What is predictive reliability?
Predictive reliability is a maintenance strategy that uses data analysis and predictive modeling techniques to forecast an equipment's performance and reliability.
Organizations can develop a predictive maintenance schedule by analyzing past failures and maintenance records to prevent disruptions and improve reliability.
It’s an essential aspect of asset management, enabling companies to identify potential issues before they occur and take preventive measures.
A computerized maintenance management system (CMMS) helps with a centralized platform for storing and analyzing performance and maintenance history.
Attaching sensor device equipment helps collect real-time data on the machine's condition. This is then streamed directly into your CMMS software, allowing you to monitor equipment status.
When sensor data indicate there’s a problem, work order are automatically generated. This means you can act before a breakdown occurs. Catching issues before they become major problems help avoid downtimes.
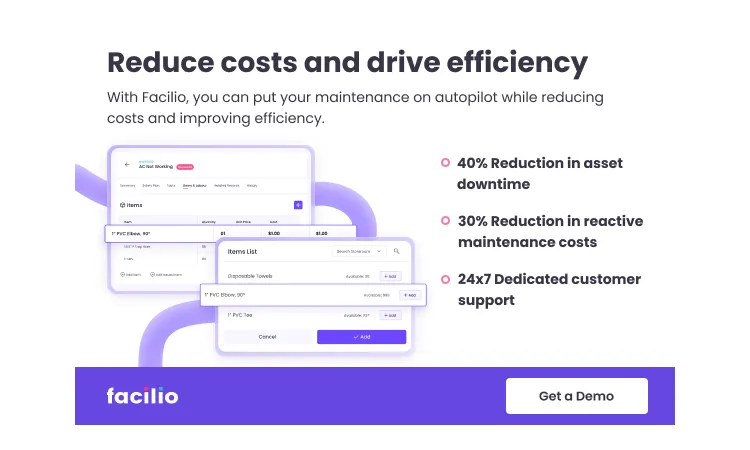
Goal of predictive reliability analysis
Predictive reliability analysis helps identify potential failures in equipment or systems before they occur and implement proactive maintenance strategies.
Businesses can achieve this through various methods, including analyzing the performance and maintenance history of the equipment, forecasting future performance and reliability using statistical analysis and predictive modeling techniques, and developing strategies.
Organizations can reduce downtime, improve productivity, and increase overall operational reliability by using data-driven approaches.
How to implement predictive reliability analysis
Implementing predictive reliability analysis in your organization can be complex.
These five steps help implement predictive reliability analysis in your organization.
1. Identify the equipment
The first step in implementing predictive reliability analysis is identifying the equipment or systems that will be evaluated. This includes anything from manufacturing machinery to IT systems to transportation equipment.
2. Develop a failure mode and effects analysis (FMEA)
FMEA is a risk assessment tool that helps organizations assess potential risks by identifying and prioritizing failure modes. To conduct FMEA, you examine each component of the system to determine possible failures and assign ratings based on probability, severity, and detection method.
This proactive identification of potential failures streamlines maintenance efforts and increases reliability.
3. Performance and maintenance history data
Gather data on equipment's performance and maintenance to predict and plan for future performance and reliability.
This helps improve your understanding of the equipment's overall reliability and identify potential issues. Collecting data over a longer period gives you a more accurate picture of the equipment's performance and maintenance needs.
4. Use statistical analysis and predictive modeling techniques
Once you have gathered the necessary data, you can use statistical analysis and predictive modeling techniques to forecast future performance and reliability.
Statistical analysis helps you understand the relationships between variables and their impact on performance and reliability.
Predictive modeling techniques allow you to forecast future performance and reliability by creating machine learning algorithms.
5. Develop and implement strategies for improving performance and reliability
One strategy is to invest in preventive maintenance, which includes regular inspections and maintenance and using advanced technologies such as vibration analysis or thermal imaging.
Another strategy is implementing new technologies that enhance performance and reliability, such as upgrading to more efficient motors or using sensors to monitor equipment performance.
You can also implement process improvements to increase performance and reliability, including streamlining production processes, standardizing operating procedures, or implementing Lean principles.
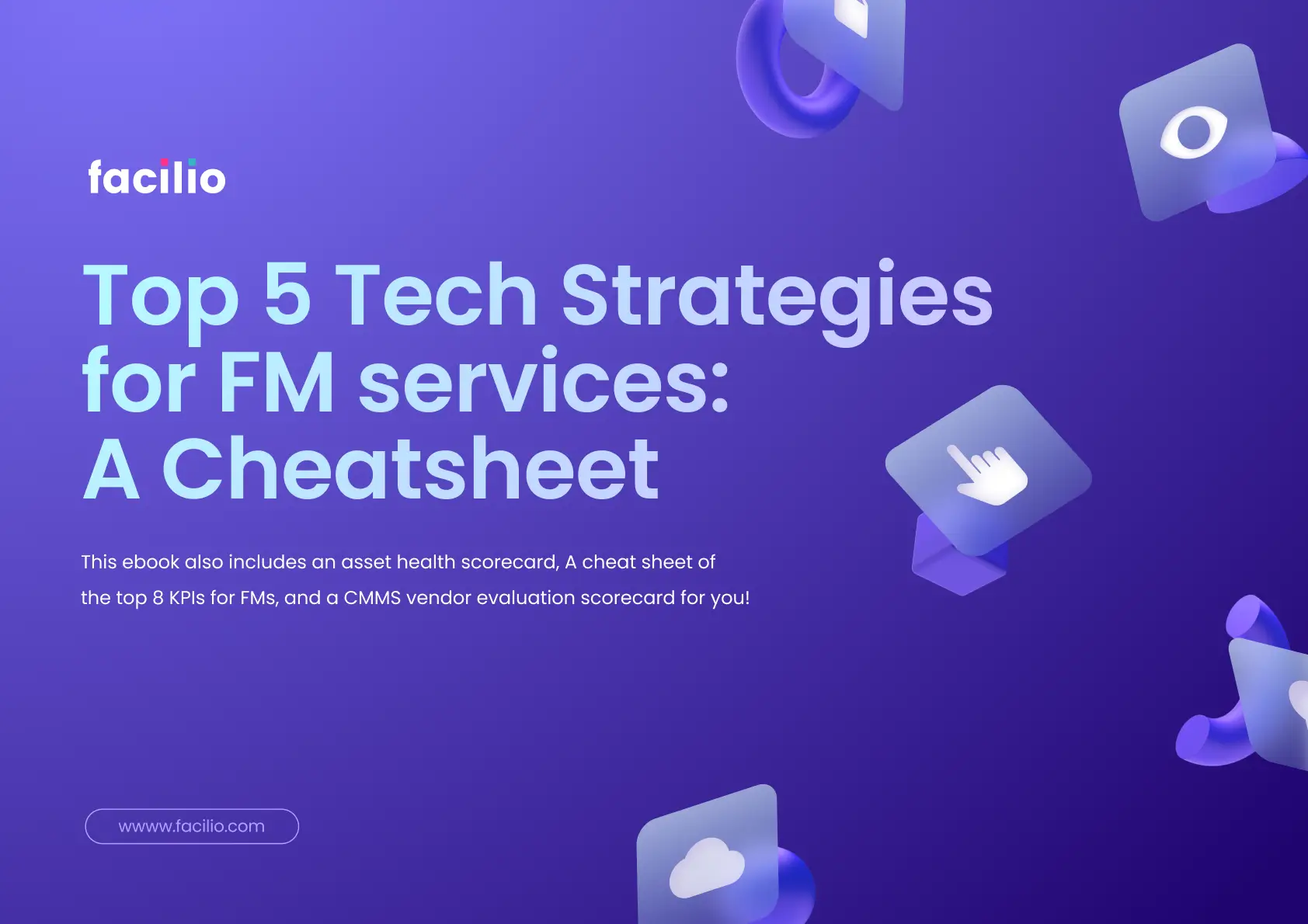
Examples of predictive reliability analysis by industry
Here are a few examples of how different industries use predictive reliability:
- Manufacturing: By analyzing data from sensors, controllers, and other sources, manufacturers can identify potential failures before they happen and schedule maintenance.
- Energy: Predictive reliability helps energy companies identify potential problems with their equipment and mitigate risks. For example, an energy company uses predictive analysis to determine when a transformer will fail and schedule maintenance or replacement before it causes an outage.
- Transportation: Predictive reliability helps these companies identify when a vehicle is likely to fail and schedule maintenance or replacement. For example, a trucking company uses predictive analysis to identify when a truck's tires are likely to wear out and replace them before they cause a breakdown.
- Healthcare: By analyzing data from sensors, controllers, and other sources, healthcare providers can identify potential failures before they occur and schedule maintenance. For example, a hospital can determine when a ventilator will fail and schedule maintenance or replacement.
Benefits of predictive reliability analysis in maintenance management
- Reduces system downtime. Organizations can prevent disruptions and minimize downtime by identifying failures in advance.
- Improves equipment reliability. Predictive reliability analysis helps organizations identify and address potential failures before they occur.
- Saves cost. Predictive analytics helps extend the lifespan of assets by identifying and fixing problems before they become major issues.
- Enhances workplace safety. Predictive reliability analysis help identifies and prevent workplace failures that may pose a safety risk.
- Helps collect continuous data. Use predictive analytics to generate real-time reports on company spending and create enhanced forecasts for future expenditures. Integrating your CMMS with HR software provides on-demand dashboards that show a complete view of human, asset, and financial capital.
- Improves asset retirement processes. Predictive analytics improves asset retirement processes by identifying when an asset nears replacement, allowing teams to sell the used but still functioning machine.
Advanced tips to help you improve your predictive reliability analysis program:
- Use machine learning and artificial intelligence. By automating the data collection and analysis process, you can more quickly and accurately identify patterns and trends in your data. This can provide valuable insights into the performance of your systems and equipment.
- Develop a comprehensive predictive maintenance strategy. In addition to predictive reliability analysis, consider incorporating other techniques, such as condition-based monitoring and spare parts management, into your strategy. This can help you prevent failures and maintain the reliability of your operations more effectively.
- Collaborate with other organizations and industry experts. Sharing data and best practices with others can help you learn from their experiences and improve the effectiveness of your program.
- Invest in training and education. Ensuring your maintenance staff has the skills and knowledge to implement and maintain a successful predictive reliability analysis program is crucial. Invest in training and education to ensure your team is well-equipped to handle this vital task.
- Continuously monitor and evaluate. Regularly monitoring and evaluating your predictive reliability analysis program can help you identify any areas for improvement. This can help you stay on top of new developments in the field and ensure that your algorithms remain effective over time.
How does CMMS help with predictive reliability analysis?
CMMS software helps organizations collect and analyze equipment performance and maintenance history data, enabling them to forecast the future reliability of their systems and equipment.
Some specific ways in which a CMMS can support predictive reliability analysis include:
- Data collection and analysis: A CMMS can automate the data collection and analysis process, making it easier to gather and analyze data on past failures and maintenance activities. This can help organizations identify patterns and trends in their data and provide valuable insights into the performance of their equipment.
- Predictive maintenance scheduling: This can help ensure maintenance is performed at the right time, on the right equipment, and by the right personnel.
- Asset management: A CMMS helps organizations track and manage their assets, including equipment and systems, enabling them to monitor performance and identify potential failures.
- Work order management: A CMMS helps track and manage work orders related to predictive maintenance activities, enabling them to optimize the effectiveness of their program.
Get in touch.
Learn how a connected CMMS solution helps.